NumerSense
Probing Numerical Commonsense Knowledge of Pre-trained Language ModelsBill Yuchen Lin, Seyeon Lee, Rahul Khanna, Xiang Ren
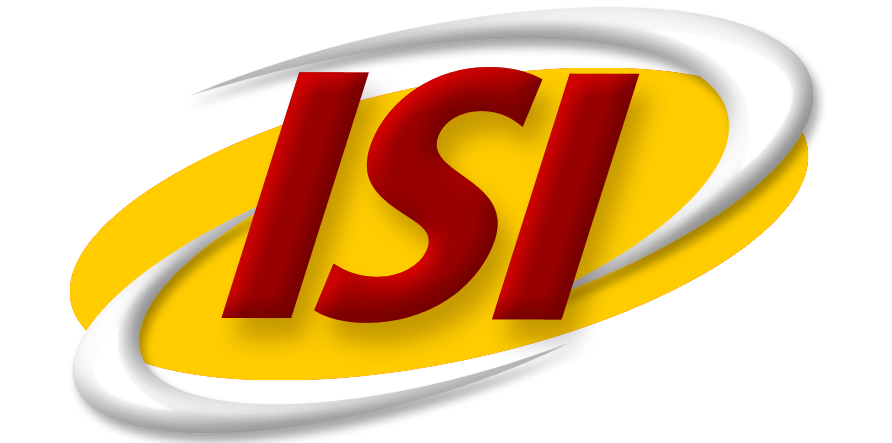
NumerSense is a new numerical commonsense reasoning probing task, with a diagnostic dataset consisting of 3,145 masked-word-prediction probes.
We propose to study whether numerical commonsense knowledge can be induced from pre-trained language models like BERT, and to what extent this access to knowledge robust against adversarial examples is. We hope this will be beneficial for tasks such as knowledge base completion and open-domain question answering.
For submitting your prediction and check the lastest submissions, please check it at the eval.ai.
Rank | Model | Hit@1 | Hit@2 | Hit@3 |
---|---|---|---|---|
|
Human Performance |
88.3 (closed-book) 93.7 (open-book) |
N/A | N/A |
1 |
University of Washington - 2021-9 |
72.47 | 85.57 | 91.58 |
2 |
ISI Waltham - 2021-04 |
66.18 | 82.80 | 89.64 |
3 |
MOWGLI/USC INK - Jun Yan - 2021-01-10 |
65.10 | 81.56 | 88.33 |
4 |
Stanford - Yuhui Zhang - 2021-01-08 |
64.08 | 79.66 | 87.29 |
5 |
Team Cosmic - Yizhong Wang - 2021-01-11 |
56.91 | 72.01 | 80.51 |
6 |
MICS ISI - Dong-Ho Lee - 2021-01-20 |
56.33 | 73.30 | 82.33 |
7 |
RoBERTa-Large (Fine-tuned) |
47.58 | 66.34 | 76.74 |
8 |
BERT-Large (Fine-tuned) |
43.68 | 66.41 | 72.87 |
9 |
RoBERTa-Large (Zero-shot) |
35.89 | 58.07 | 74.09 |
10 |
BERT-Large (Zero-shot) |
27.15 | 52.92 | 70.25 |
11 |
RoBERTa-base (Zero-shot) |
26.80 | 50.57 | 66.72 |
12 |
BERT-base (Zero-shot) |
25.30 | 48.70 | 64.84 |
13 |
GPT-2 (Zero-shot) |
24.76 | 44.28 | 62.40 |
Rank | Model | Hit@1 | Hit@2 | Hit@3 |
---|---|---|---|---|
|
Human Performance |
89.7 (closed-book) 96.3 (open-book) |
N/A | N/A |
1 |
University of Washington - 2021-9 |
79.24 | 89.93 | 94.17 |
2 |
ISI Waltham - 2021-04 |
72.61 | 87.10 | 92.23 |
3 |
MOWGLI/USC INK - Jun Yan - 2021-01-10 |
70.41 | 84.81 | 90.99 |
4 |
Stanford - Yuhui Zhang - 2021-01-08 |
70.23 | 83.57 | 90.11 |
5 |
Team Cosmic - Yizhong Wang - 2021-01-11 |
62.51 | 75.77 | 82.40 |
6 |
MICS ISI - Dong-Ho Lee - 2021-01-20 |
60.87 | 76.33 | 84.54 |
7 |
RoBERTa-Large (Fine-tuned) |
54.22 | 69.53 | 78.97 |
8 |
BERT-Large (Fine-tuned) |
50.19 | 66.23 | 74.72 |
9 |
RoBERTa-Large (Zero-shot) |
46.11 | 66.08 | 79.42 |
10 |
BERT-Large (Zero-shot) |
37.54 | 62.10 | 76.86 |
11 |
RoBERTa-base (Zero-shot) |
33.39 | 58.83 | 71.91 |
12 |
BERT-base (Zero-shot) |
31.98 | 56.01 | 70.67 |
13 |
GPT-2 (Zero-shot) |
30.04 | 51.06 | 67.58 |
@inproceedings{lin2020numersense,
title={Birds have four legs?! NumerSense: Probing Numerical Commonsense Knowledge of Pre-trained Language Models},
author={Bill Yuchen Lin and Seyeon Lee and Rahul Khanna and Xiang Ren},
booktitle={Proceedings of EMNLP},
year={2020},
note={to appear}
}